Dobb·E
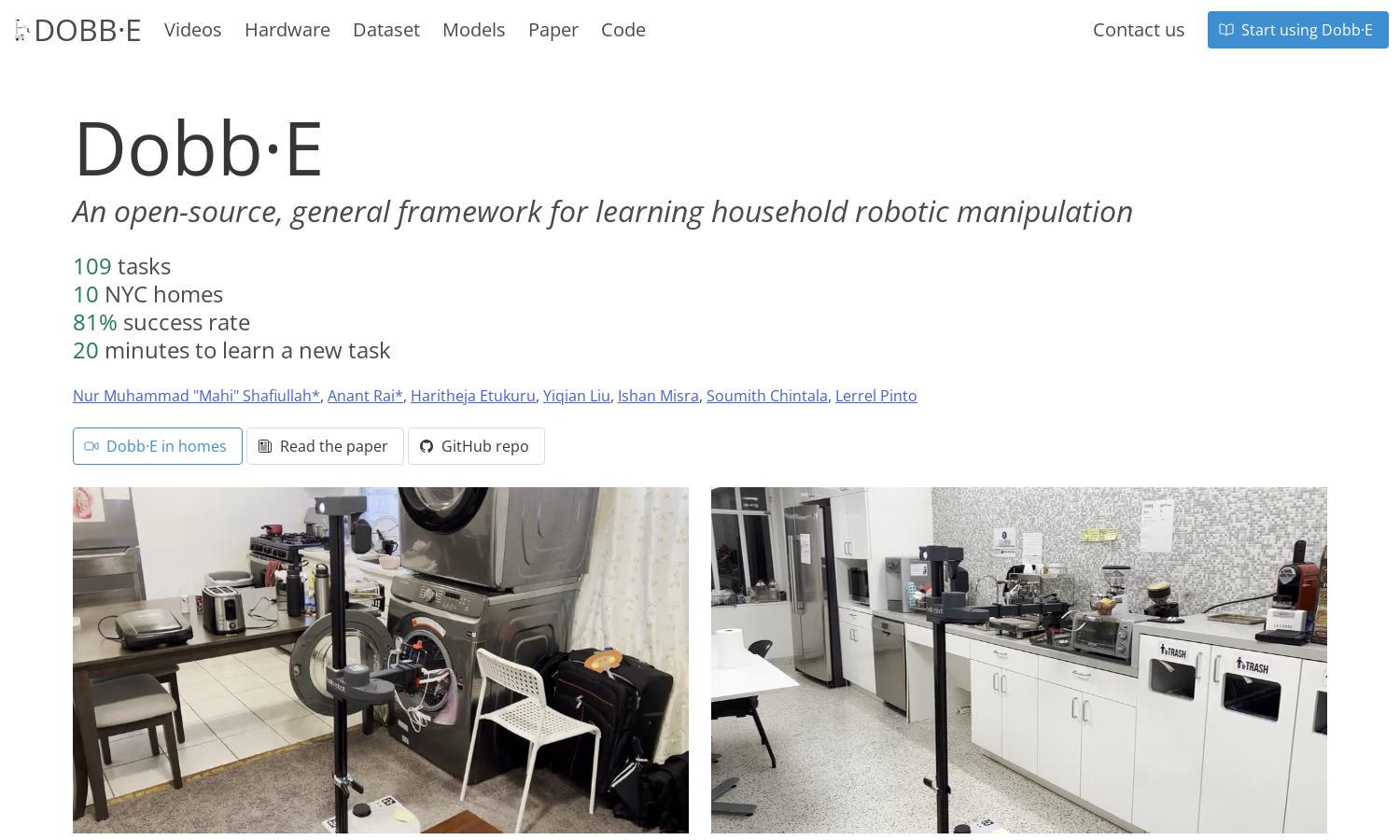
About Dobb·E
Dobb·E is a groundbreaking open-source framework that equips robots to learn household tasks rapidly through imitation learning. With a unique tool called "The Stick" for data collection and a robust dataset, Dobb·E enables real-time task adaptation, benefiting households with versatile robotic helpers.
Dobb·E offers an open-source solution for $0, making it accessible for research and home robotics. Users can leverage a variety of datasets and models, enhancing their robotic systems' efficiency and capabilities without financial burden, promoting innovation in home automation.
Dobb·E features an intuitive interface that simplifies user interaction and navigation. Its clean design enhances the learning experience, enabling seamless access to tools and resources, which optimizes the process of teaching robots new tasks, making robotics more user-friendly.
How Dobb·E works
Users begin by utilizing Dobb·E's demonstration tool, "The Stick," to collect five minutes of task demonstrations. Following this, Dobb·E's machine learning algorithms adapt to the new environment using data from its extensive dataset, enabling the robot to execute a novel task with an impressive average success rate of 81%.
Key Features for Dobb·E
Imitation Learning
Dobb·E employs a unique imitation learning approach, allowing robots to learn tasks effectively through minimal user interaction. This innovative method significantly reduces the time needed for teaching robots, offering a reliable solution for efficient home automation.
Homes of New York Dataset
The Homes of New York (HoNY) dataset is crucial for training Dobb·E, as it provides over 13 hours of diverse interactions in real homes. This rich dataset enhances the model's ability to adapt to various household environments, ensuring reliability and efficiency in task execution.
Home Pretrained Representations (HPR)
HPR serves as a foundational model pre-trained on the HoNY dataset, enabling robots to quickly adapt and perform new tasks in unfamiliar environments. This pre-training accelerates the learning process, increasing the overall effectiveness of robots in household applications.
You may also like:
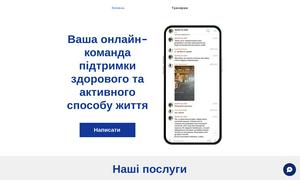